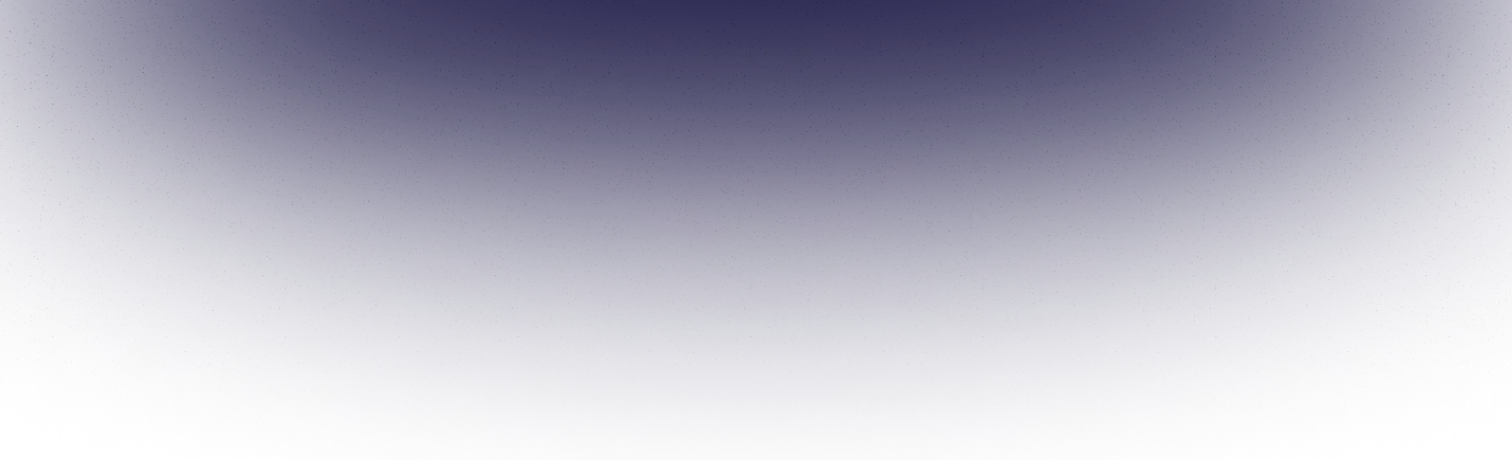
What is the difference between AI and Generative AI?
Artificial Intelligence (AI) is a rapidly evolving field that has gained significant attention in recent years. It encompasses various technologies and techniques that enable machines to mimic human intelligence and perform tasks with varying degrees of autonomy. Within the broader domain of AI, there exists a specific branch known as Generative AI. In this blog post, we will delve into the differences between AI and Generative AI, shedding light on their distinct characteristics and applications. By understanding these differences, we can appreciate the unique capabilities and potential of Generative AI.
Importance of artificial intelligence (AI) in various domainsArtificial Intelligence (AI) has emerged as a transformative technology with the potential to revolutionize various domains and industries. It encompasses a range of techniques and algorithms that enable machines to perform tasks that typically require human intelligence. From healthcare to finance, transportation to agriculture, AI is making significant contributions by automating processes, analyzing vast amounts of data, and generating valuable insights. In this blog post, we will explore the importance of AI in various domains and the impact it is having on the way we live and work.
- Healthcare: AI is revolutionizing healthcare by improving diagnosis, treatment, and patient care. Machine learning algorithms can analyze medical images, such as X-rays and MRIs, to assist in early detection of diseases and provide more accurate diagnoses. AI-powered systems can also predict patient outcomes and recommend personalized treatment plans based on medical records and genetic data. Additionally, chatbots and virtual assistants are being employed to provide round-the-clock support and answer basic medical questions, enhancing access to healthcare services.
- Finance: AI is transforming the financial industry by automating tasks, detecting fraud, and making data-driven decisions. Natural Language Processing (NLP) algorithms can analyze news articles and social media data to predict market trends and optimize investment strategies. Machine learning models can assess creditworthiness, detect anomalies in transactions, and identify potential fraudulent activities. AI-powered chatbots and virtual assistants are also enhancing customer service by providing personalized recommendations and assistance with financial queries.
- Transportation: AI is playing a crucial role in improving transportation systems, making them safer, more efficient, and environmentally friendly. Autonomous vehicles, enabled by AI algorithms and sensors, have the potential to reduce accidents and congestion while optimizing fuel consumption. AI-powered route optimization systems can optimize delivery routes, reducing costs and minimizing travel time. Furthermore, AI is used in traffic management to analyze real-time data and make informed decisions for traffic control and signal optimization.
- Agriculture: AI is revolutionizing agriculture by enhancing crop management, yield prediction, and resource optimization. Machine learning algorithms can analyze satellite imagery, weather data, and soil conditions to optimize irrigation, fertilization, and pest control. AI-powered drones and robots can monitor crops, identify diseases, and perform precise spraying or harvesting operations. These advancements help improve crop yields, reduce resource waste, and contribute to sustainable agriculture practices.
- Customer Service: AI is transforming customer service by providing personalized and efficient support. Natural Language Processing algorithms enable chatbots and virtual assistants to understand and respond to customer queries, providing instant assistance. AI-powered sentiment analysis can analyze customer feedback and sentiments to identify areas for improvement and enhance customer satisfaction. Additionally, AI algorithms can analyze customer preferences and behavior to offer personalized product recommendations and enhance the overall customer experience.
The importance of artificial intelligence in various domains cannot be overstated. AI is revolutionizing healthcare by improving diagnosis and treatment, enhancing finance by automating processes and detecting fraud, transforming transportation by optimizing routes and enabling autonomous vehicles, revolutionizing agriculture by improving crop management and resource optimization and enhancing customer service by providing personalized support and recommendations. As AI continues to advance, it will unlock new possibilities, drive innovation, and shape the future of numerous industries, ultimately improving our lives and creating a more efficient and intelligent world.
Understanding Artificial Intelligence (AI)
Artificial Intelligence (AI) is a transformative technology that has gained significant attention in recent years. It refers to the development of intelligent machines that can perform tasks that typically require human intelligence. AI encompasses various techniques and approaches, including machine learning, which enables computers to learn from data and improve their performance over time. In this blog post, we will explore the fundamentals of AI, including its definition, different types of AI, and examples of its applications across various industries.
What is AI, and what are the different types of AI?
Artificial Intelligence (AI) is the field of computer science that focuses on creating intelligent machines capable of mimicking human cognitive functions. It involves the development of algorithms and systems that can perceive and understand information, reason, learn, and make decisions.
- Narrow AI: Also known as weak AI, narrow AI refers to AI systems that are designed to perform specific tasks or functions. These systems excel in a particular domain but lack the ability to generalize beyond their predefined tasks. Examples of narrow AI include voice assistants, image recognition systems, and recommendation algorithms.
- General AI: General AI, also referred to as strong AI or artificial general intelligence aims to develop machines that possess the ability to understand, learn, and perform any intellectual task that a human being can do. General AI systems would exhibit human-level intelligence and flexibility, enabling them to adapt to various situations and learn new tasks.
- Machine Learning: Machine learning is a subset of AI that focuses on the development of algorithms and statistical models that enable computers to learn from data and improve their performance without being explicitly programmed. Machine learning algorithms can identify patterns, make predictions, and automate complex tasks by analyzing large datasets.
Examples of AI applications in various industries
AI has found applications across numerous industries, revolutionizing the way tasks are performed and generating valuable insights. Here are a few examples:
- Healthcare: AI is used for medical image analysis, diagnosis, drug discovery, and personalized medicine.
- Finance: AI powers fraud detection, risk assessment, algorithmic trading, and customer service in the financial industry.
- Transportation: AI is employed in autonomous vehicles, traffic management, route optimization, and predictive maintenance.
- Retail: AI is used for demand forecasting, personalized marketing, chatbots for customer service, and inventory management.
- Manufacturing: AI enables predictive maintenance, quality control, process optimization, and robotics automation.
Artificial Intelligence encompasses the development of intelligent machines capable of performing tasks that typically require human intelligence. It includes narrow AI, which focuses on specific tasks, and general AI, which aims for human-level intelligence. Machine learning is a subset of AI that enables computers to learn from data and improve their performance. AI has significant applications across industries, from healthcare and finance to transportation and retail, revolutionizing processes, improving efficiency, and generating valuable insights. Understanding the fundamentals of AI and its diverse applications is crucial in navigating the rapidly evolving technological landscape and harnessing its potential for innovation and problem-solving.
Exploring Generative AI
Generative AI is a subset of artificial intelligence (AI) that focuses on the creation and generation of new content, such as images, text, and sounds, that closely resembles existing data. Unlike other types of AI that primarily focus on recognizing patterns or making predictions, generative AI models are designed to produce novel and realistic outputs based on the patterns and structures learned from training data. In this blog post, we will explore the fundamentals of generative AI, including its definition, key characteristics, and how it differs from other types of AI.
What are Generative AI and what Key characteristics and features of generative AI?
Generative AI refers to the field of AI that involves the development of models and algorithms capable of generating new content that is similar to a given dataset. These models learn the underlying patterns and structures of the training data and use that knowledge to create new and original content. Key characteristics of generative AI include:
- Creativity: Generative AI models have the ability to create content that does not exist in the original dataset. They can produce diverse and unique outputs by leveraging learned patterns and generating variations.
- Realism: Generative AI aims to generate content that is realistic and resembles the training data. Whether it is generating images, text, or music, the outputs should be coherent and convincing to human observers.
- Learning from Data: Generative AI models learn from large datasets and extract complex patterns and dependencies. They use this learned knowledge to generate new content by applying those patterns and relationships.
Distinction between generative AI and other types of AI
Generative AI differs from other types of AI, such as discriminative AI or predictive AI, in terms of their primary objectives and approaches.
- Discriminative AI: Discriminative AI models focus on classifying or differentiating inputs into specific categories or classes. They aim to find the boundary or decision boundary that separates different classes in the data. Examples include image classification or sentiment analysis models that classify inputs into predefined categories.
- Predictive AI: Predictive AI models aim to make predictions or forecasts based on existing data. They analyze patterns and relationships in the data to predict future outcomes. Examples include time series forecasting models or recommendation systems that predict user preferences.
Generative AI, on the other hand, is focused on generating new and original content that is similar to the training data. It leverages the learned patterns and structures to create novel outputs rather than classifying or predicting existing data.Generative AI is an exciting field within the broader realm of artificial intelligence. It involves the development of models and algorithms that can generate new and realistic content based on the patterns and structures learned from training data. Key characteristics of generative AI include creativity, realism, and the ability to learn from data. It is distinct from other types of AI that focus on classification or prediction. By understanding the fundamentals of generative AI and its unique features, we can explore its potential applications in various domains, such as art, music, design, and data augmentation, and unlock new possibilities for creative content generation and problem-solving.
AI vs. Generative AI: The Core Differences
The following are the core differences between AI and Generative AI:AspectAIGenerative AIFocusProblem-solving and decision-makingCreation of new and unique contentTraining DataRelies on labeled data for supervised learning OutputLimited to predefined outputs and actionsGenerates new and diverse outputsExamplesSpeech recognition, recommendation systemsImage synthesis, text generation, music compositionEmphasisTask-specific solutionsNovelty and creativityIn the above table, we compare AI and Generative AI in terms of their focus, training data, output, examples, and emphasis. AI primarily focuses on problem-solving and decision-making tasks, utilizing labeled data for supervised learning. On the other hand, Generative AI places emphasis on the creation of new and unique content, employing unsupervised learning techniques. Furthermore, AI's output is often limited to predefined outputs and actions based on the training data, while Generative AI has the capability to generate new and diverse outputs that go beyond the limitations of the training data. Examples of AI applications include speech recognition and recommendation systems, while Generative AI finds applications in image synthesis, text generation, and music composition, among others.
Implications and Future Possibilities of the Difference between AI and Generative AI
The difference between AI and Generative AI opens up a range of implications and future possibilities across various domains. While AI focuses on problem-solving and decision-making tasks, Generative AI excels at creating new and unique content. Understanding these distinctions allows us to explore the potential impact and advancements that can be achieved in fields such as creativity, personalization, content generation, and data synthesis.
- Enhanced Creativity and Innovation: Generative AI empowers us with tools to push the boundaries of creativity and innovation. By generating new and unique content, it opens up possibilities for artists, designers, and creators to explore novel ideas and expand their creative horizons. The ability to generate content that goes beyond what already exists can lead to the discovery of new artistic styles, music genres, and design concepts.
- Personalized User Experiences: With Generative AI, personalized user experiences can be taken to new heights. By leveraging generative models, applications can adapt and tailor their outputs based on individual preferences and characteristics. This could be in the form of personalized recommendations, customized products, or interactive virtual assistants that understand and respond to users in a more personalized and engaging manner.
- Content Generation and Automation: Generative AI has the potential to transform content creation and automation. With the ability to generate content such as images, videos, and text, it can automate the production of large volumes of content, saving time and resources. This can be particularly useful in areas such as marketing, advertising, and media production, where a constant stream of engaging and diverse content is required.
- Synthetic Data Generation: Generative AI can also play a crucial role in synthetic data generation. It can generate synthetic datasets that mimic real-world data, allowing for extensive training and testing of AI models. Synthetic data generation helps overcome limitations in data availability and privacy concerns while providing a diverse and controlled dataset for training and validation purposes.
Conclusion
In conclusion, the distinction between AI and generative AI highlights the unique capabilities and possibilities offered by generative AI models. While AI focuses on problem-solving and decision-making tasks, generative AI excels at creating new and unique content. Understanding these differences is crucial as it opens up a world of opportunities and advancements across various domains. By recognizing the distinctions between AI and generative AI, we can fully appreciate the power of generative AI in enhancing creativity, personalization, content generation, and synthetic data generation. The ability to generate new and unique content pushes the boundaries of innovation and opens doors for artists, designers, and creators to explore novel ideas.
The potential of generative AI in personalized user experiences cannot be overstated. By tailoring outputs based on individual preferences, it enhances user engagement and satisfaction. Additionally, generative AI enables the efficient content generation and automation, saving time and resources in industries that require a constant stream of diverse and engaging content.Furthermore, generative AI plays a crucial role in synthetic data generation, providing a controlled and diverse dataset for training and validation. This addresses data availability and privacy concerns while ensuring the development and testing of robust AI models.
In light of these possibilities, it is essential for readers to explore the potential of generative AI in their respective fields. By embracing generative AI techniques and tools, individuals and organizations can unlock new avenues for creativity, personalization, and efficiency.In conclusion, the distinction between AI and generative AI provides valuable insights into the capabilities and potential of generative AI models. By understanding and harnessing generative AI, we can drive innovation, enhance user experiences, streamline content generation, and leverage synthetic data for improved AI applications. It is an exciting time to explore and embrace the possibilities that generative AI has to offer.
Partner with PixelBin.io to Set your Business Standards
Small business owners frequently need to manage, edit, create, and optimize multiple images. PixelBin is the nimble, one-stop solution to simplify your image-related tasks with ease so that you can focus on business growth.
Ready to give it a try?