Shaping the Future of eCommerce with Image Segmentation


Living in a digital world, we find that images communicate more effectively than words and everything that you see on your screen is directly linked to the decisions a customer might or might not make. Amidst this shift, image segmentation stands out as an industry game-changer. It shapes the future of eCommerce by segmenting the visuals as desired, personalizing the user experience to the core.
β
Providing unparalleled precision and understanding of visual data brings revolutionary changes to various industries. Forecasts predict the global image segmentation market up to a staggering $15.5 billion by 2026, demonstrating the tremendous value and demand for advancements in technology.
β
What is Image Segmentation? Why should we care?
Take a crowded street photograph as an example.
Picture the capability to accurately identify and separate each person, vehicle, and object in that image. That's the essence of image segmentation. This process partitions an image into distinct and meaningful regions, assigning each pixel to a specific category or class. This ability to unravel an image's intricate boundaries and structures unlocks countless possibilities, from refining computer vision systems to facilitating advanced image manipulation techniques.
β
- The application of image segmentation is pivotal in the healthcare sector, particularly in medical imaging analysis. Doctors can accurately locate and diagnose abnormalities by segmenting medical images like MRI scans or X-rays. This results in timely treatment and better results.
β
- In the retail and eCommerce sectors, image segmentation boosts object detection and recognition, enabling automated inventory management, personalized marketing, auto-image tagging, and an effortless user experience.
β
So, let us understand how does image segmentation work in practice?
Which key techniques and algorithms does it use?
What recent advancements have pushed the boundaries in this field?
We'll further look at the challenges researchers and practitioners face when implementing image segmentation algorithms and PixelBin's performance using image segmentation to serve its eCommerce clients.
β
Understanding Image Segmentation Techniques
Image segmentation is an effective technique, allowing us to analyze and comprehend images accurately. We can dissect an image and examine its various parts using effective processes and algorithms.
β
- One prevalent technique is "Thresholding". It groups pixels based on their brightness or darkness. By defining a particular brightness level, we can separate brighter or darker pixels from this level into distinct groups. This separation allows us to isolate the objects we want to study.
β
- Another technique, "Region-based Segmentation", groups pixels based on similarities in color, texture, or other visual properties. Algorithms like "K-means clustering" and "Mean-Shift" divide the image into distinct regions with similar characteristics. These algorithms examine the properties of neighboring pixels to detect boundaries between different objects and separate them effectively.
β
- "Graph-based Segmentation" algorithms apply the concept of graphs to partition an image into areas. Each pixel is a point in a graph, and their relationships form their connections. Algorithms like "Normalized Cut" or "Watershed" divide the graph into meaningful segments, considering local and global information. This technique is beneficial for images with complex structures or varied textures.
β
- Furthermore, "Deep Learning" techniques like Convolutional Neural Networks (CNNs) have revolutionized image segmentation. Models like "U-Net" and "Mask R-CNN" employ deep neural networks to learn intricate patterns and relationships within images, yielding exact pixel-level segmentation. These models learn from large sets of labeled data and can adaptively capture complex features, making them a popular choice across various fields.
β
As image segmentation evolves, advanced algorithms like "Graph Cuts", "Level Sets", and "Active Contours" continue to develop, offering superior results in complex scenarios. Researchers are also investigating hybrid approaches that combine multiple techniques, such as "Graph Cut with Deep Learning", to leverage the strengths of different methods and achieve superior segmentation performance.
β
These varied techniques and algorithms continuously push the boundaries of computer vision, enabling us to identify objects accurately, understand scenes, and manipulate images. By leveraging these advanced tools, researchers and professionals can unlock new realms of visual analysis and explore exciting applications across various domains.
β
Recent Advancements in Image Segmentation
- Augmented Reality: Image segmentation is vital in crafting engaging augmented reality experiences. Consider a smartphone app that places virtual objects in your surroundings. Image segmentation allows the app to differentiate between the foreground (such as your body or face) and the background environment. This division helps integrate virtual objects seamlessly with the real world.
β
- Fashion and Retail: Tasks like garment segmentation and body measurement analysis in the fashion and retail industry employ image segmentation. It facilitates virtual try-on experiences where customers can visualize how clothing items would look on them without physically trying them on. By segmenting an image of a person, we can overlay the virtual garment accurately on their body, offering a true-to-life representation.
β
Early Adoptions of Image Segmentation
Medical imaging was one of the first fields to embrace image segmentation, recognizing its potential to aid in diagnosis and treatment planning. They revolutionized healthcare by separating anatomical structures and abnormalities in X-rays and CT scans. At the same time, researchers in robotics realized the importance of image segmentation for autonomous navigation.
β
By segmenting visual input from cameras on robots, they could distinguish obstacles from the environment, leading to advancements in autonomous vehicles, drones, and interactive robots. Image segmentation also found applications in geology and agriculture. Geologists used it to analyze satellite images for terrain features, land cover mapping, and geological analysis. Beyond traditional fields, image segmentation became a tool for artists, designers, and the advertising industry.
β
It enabled stunning visual effects, image manipulation, precise audience targeting, and personalized content delivery. These early adoptions set the stage for image segmentation's widespread use today. With technological advancements, more sophisticated algorithms and techniques refined the segmentation process and expanded its applications.
β
From improving medical diagnoses to enabling autonomous vehicles, image segmentation continues to shape how we perceive and interact with visual data. The visionary pioneers who adopted image segmentation transformed industries and pushed the boundaries of what was possible. Here, we have accumulated a few requirements for the successful execution of image segmentation at the researchers' end.
β
Requirements for Successful Image Segmentation
Successful image segmentation requires meeting essential requirements to achieve accurate and reliable results. Each aspect plays a critical role in the segmentation process, from the careful selection of algorithms to the availability of diverse datasets.
β
By understanding and fulfilling these requirements, practitioners and researchers can unlock the full potential of image segmentation, enabling precise object recognition, scene understanding, and enhanced visual analysis. Here, we have listed the critical requirements for successful image segmentation, highlighting their significance and impact on achieving optimal segmentation outcomes.
β
- Diverse and Representative Dataset: A comprehensive dataset with varied images, capturing different scenarios, objects, and backgrounds, allows the segmentation model to learn and adapt to various visual contexts. Annotated ground truth data provides essential training and evaluation material.
β
- Appropriate Segmentation Algorithms and Techniques: The selection of suitable algorithms, such as thresholding, region-based segmentation, or deep learning models, depends on the specific image and application requirements. Understanding the strengths and limitations of each technique ensures effective segmentation.
β
- Sufficient Computational Resources: Adequate processing power and memory capacity are necessary for efficient and real-time segmentation. High-performance hardware, like GPUs, accelerates the computational process, particularly for large-scale datasets.
β
- Domain Expertise and Human Intervention: Expert knowledge can guide the segmentation process, addressing challenges that automated algorithms may encounter. Human validation and refinement through interactive interfaces or post-processing techniques enhance accuracy.
β
- Continuous Learning and Adaptation: Staying updated with the latest advancements in image segmentation is crucial. Regularly exploring new algorithms, techniques, and datasets enables the refinement and improvement of segmentation models to meet evolving requirements.
β
Image Segmentation in eCommerce
β

β
eCommerce industries are harnessing the power of image segmentation to drive business growth and innovatively cater to user demands. These industries can enhance product visualization, streamline inventory management, and personalize user experiences by leveraging image segmentation techniques. Hereβs how an eCommerce business utilize image segmentation for easier workflows and extraordinary results.
β
Object Detection
Image segmentation can be used to detect and locate specific objects within product images. By segmenting the image, it becomes easier to identify individual items, such as clothing, accessories, or electronics. This enables better categorization and organization of products on eCommerce platforms.
β
Object Categorization
Additionally, image segmentation facilitates object recognition and categorization, enabling efficient inventory management. By segmenting product images based on their attributes, such as color, style, or category, eCommerce platforms can streamline catalog organization, search functionalities, filter options, and tagging. This simplifies the browsing process for customers, allowing them to find desired products more efficiently.
β
Virtual Try-on
Furthermore, image segmentation enables virtual try-on experiences, particularly in the fashion and beauty sectors. By accurately segmenting human figures and extracting key features, customers can virtually try on clothing, accessories, or makeup products, allowing them to visualize how the items would look on themselves. This immersive and interactive experience enhances user engagement and confidence in their purchase decisions.
β
Business Growth
eCommerce industries leverage image segmentation to drive business growth by improving product visualization, optimizing inventory management, and delivering personalized experiences. By harnessing the power of this technology, these industries can cater to user demands, enhance user engagement, and ultimately boost sales and customer satisfaction. As image segmentation techniques advance, we can expect even more innovative applications in eCommerce, transforming how customers interact with products online.
β
Personalization
Personalization is another crucial aspect where image segmentation plays a vital role. eCommerce platforms can deliver personalized recommendations and targeted marketing campaigns by segmenting user-generated images or analyzing user preferences. By combining image segmentation with personalized recommendations, the shopping experience can be tailored to individual customers. Here's how personalization can be incorporated:
β
- Product Recommendations: Image segmentation can be used to analyze the visual features of products and identify similar items. By segmenting images and extracting key attributes like color, pattern, or style, personalized recommendations can be generated based on the customer's browsing and purchase history. This enables eCommerce platforms to suggest relevant products that match the customer's preferences and increase the likelihood of conversion.
β
- Visual Search: Image segmentation can power visual search capabilities, allowing customers to find products by uploading or capturing images. By segmenting the uploaded image and comparing the segmented regions with the product catalog, personalized search results can be delivered based on the visual similarity of the segmented objects. This helps customers discover products that closely match their desired visual attributes.
β
- Style Matching: Image segmentation can assist in style matching by analyzing the visual characteristics of products and matching them with the customer's preferred style. By segmenting and comparing the segmented regions of product images, personalized recommendations can be provided that align with the customer's unique aesthetic preferences.
β
- User-Generated Content Analysis: Image segmentation can be applied to user-generated content, such as social media images or customer reviews. By segmenting and analyzing the visual elements within the images, personalized recommendations can be generated based on the customer's preferences and interests. This allows eCommerce platforms to leverage user-generated content to provide tailored product suggestions.
β
- Personalized Advertisements: Image segmentation can be used to personalize advertisements based on the customer's preferences and previous interactions. By segmenting product images and understanding the customer's visual preferences, targeted advertisements can be created that showcase products matching the segmented attributes of interest to the customer.
β
By incorporating personalization techniques with image segmentation, eCommerce platforms can offer a more tailored and relevant shopping experience to individual customers. This not only increases customer satisfaction but also improves engagement, conversion rates, and customer loyalty.
β
βRead this article to understand how PixelBin utilizes image segmentation technique for precise background removal.
β
How PixelBin Serves with the Best Image Quality Results in eCommerce?
PixelBin's served as a game-changer for an eCommerce giant with its Erase.bg feature, transforming their product image editing process with unparalleled precision and efficiency. While the existing Erase.bg model finely performed in removing backgrounds; it occasionally resulted in pixelated areas or resulted in unclear background removal. Our client demanded fine-tuning of the product specifically for humans to be extracted in the foreground.
β
For instance, if you compare the below images, there is a great quality difference between the result from the old model and the improved version. Our client already used Erase.bg for background removal in its regular workflow. However, they required more accuracy and sharper results for this particular case.
β
Comparing the results below, you can see that the image result has no ambiguity in the improved model. It is a clear and sharp human image extraction without any distraction involvement of wall logos, human shadows, and all intricate details that a human eye might miss.
β
.webp)
β
β
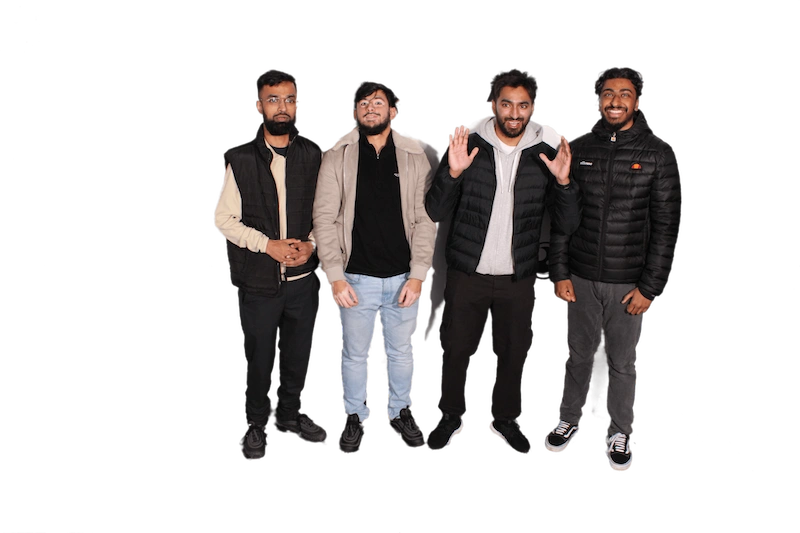
β
β
.webp)
β
PixelBin fine-tuned the model, addressing the complex pixelation issue and improving the model with sharp and precise background removal. We used image segmentation to extract human figures with precision, preserving the details and retaining the original image quality. The enhanced model leveraged this technique to effectively optimize areas where shadows were noticeably visible.
β
Vision Ahead
PixelBin's journey doesn't end there. Building upon its success in human extraction, we plan to further train the model on specific objects and props that are regularly encountered in images. This will allow the model to seamlessly remove backgrounds around these objects, enhancing overall image quality and streamlining the editing process in diverse areas.
β
Conclusion
Image segmentation is a powerful tool in computer vision, enabling precise analysis and understanding of visual data. With various techniques and algorithms, image segmentation has made significant strides in various fields, including autonomous vehicles, medical imaging, augmented reality, forensics, and retail. By separating images into meaningful regions, image segmentation facilitates object detection, scene understanding, and image manipulation, fostering innovation and problem-solving across industries.
β
Additionally, as image datasets grow and become more diverse, researchers can harness the power of deep learning and artificial intelligence to develop more accurate and adaptable segmentation models. Fusing image segmentation with other computer vision tasks, such as object tracking and 3D reconstruction, will unlock new possibilities for advanced visual analysis and understanding.
β
Looking for precise background removal?
Want to transform images in bulk?
Have a custom requirement?
Look no further than PixelBin experts to resolve all your doubts. Sign up for free today and avail exceptional benefits for business growth.